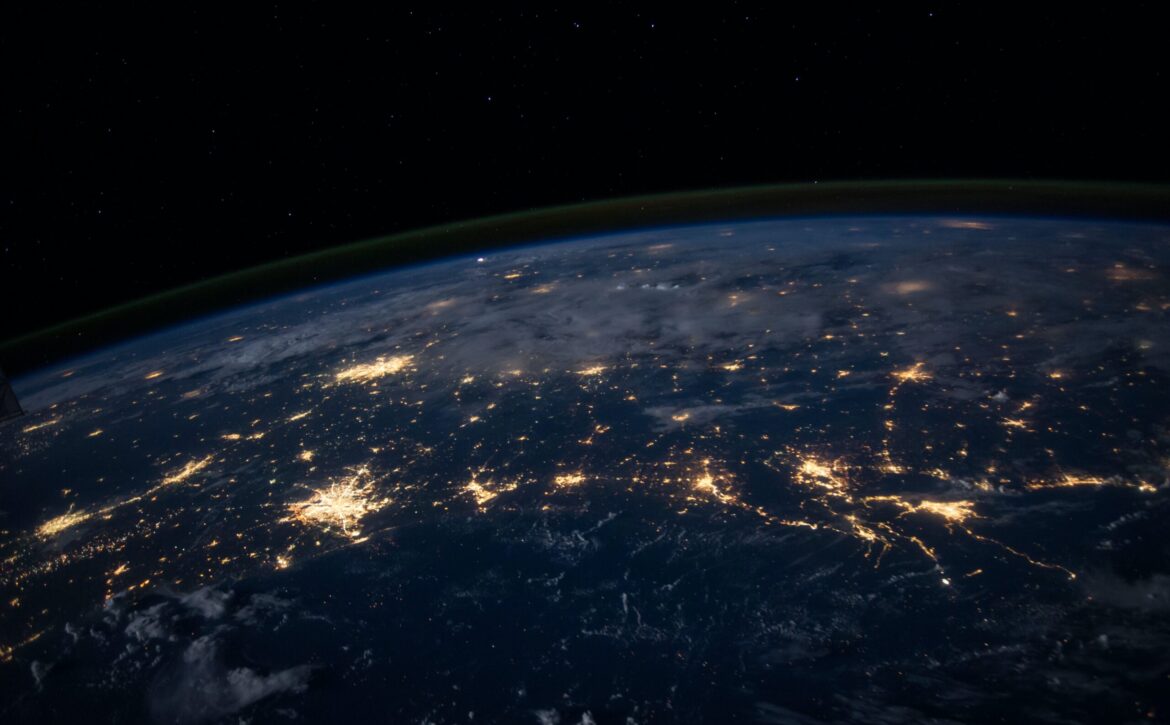
Why Responsible AI should matter?
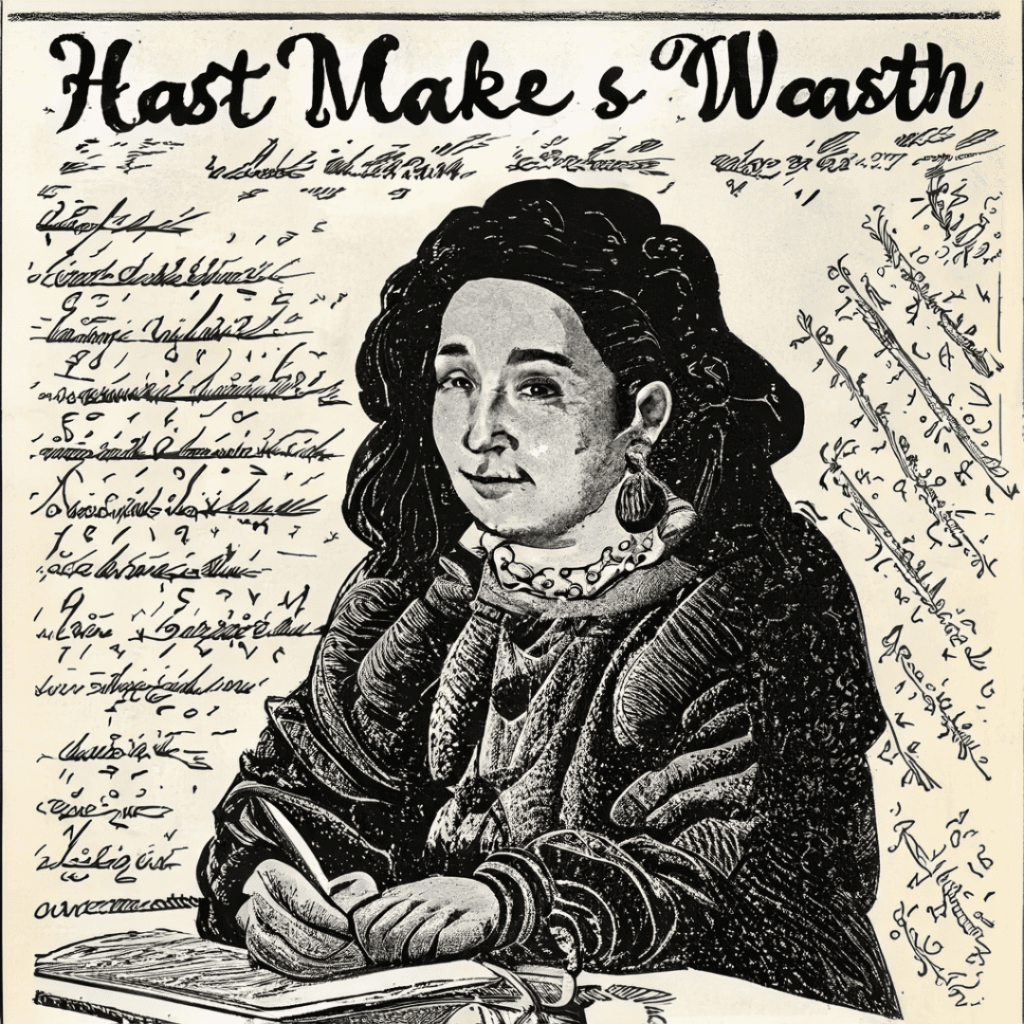
“Haste makes Waste.” – Poor Richard’s Almanack, 1753
Haste makes Irresponsible AI
When Benjamin Franklin made this famous quote, he was telling us that when we do something too quickly, it can cause mistakes that could result in something being wasted, such as time. If he had written this 270 years later, he might have written, “Haste makes Irresponsible AI”.
AI is here and we are unable to turn back the clocks. In some cases, AI is resembling some of the fears from a 1960’s sci-fi movie. It has been known to wrongly claim individuals as criminals, to drive through red lights, make racists and sexist tweets, be biased against females in recruiting, misdiagnose cancers and the list continues to grow. Is AI inherently evil? The question whether humans are inherently good or evil has been debated by Aristotle, Sigmund Freud among many others. However, with AI it is somewhat simpler.
Irresponsible AI is generally caused by either issues with the model or issues with data. The algorithms that make up a model can be generative or discriminative. A generative algorithm learns a style or pattern and generates new outputs or data (think ChatGPT), whereas discriminative algorithms take existing data to draw a conclusion (think object classification in autonomous vehicles). Discriminative AI algorithms either use classification or regression. In classification it takes inputs and puts them in specific categories, such as human vs. not a human, or heart disease vs. no heart disease. While regression estimates values and prioritizes them. Classifiers are generally more polarizing, meaning they can be really, really right, or really, really wrong. Another characteristic of a model that can cause issues is whether it is focused more on specificity or on sensitivity. Sensitive classifiers tend to create more false positives while specific classifiers tend to create more false negatives. Either could cause an issue, depending on the specific application.
Be responsible in your approach
Data is not free from causing issues. The data itself can be biased, where the training data didn’t cover all the “noise” the conditions that exist in real life. Or data can drift, where the real data is different than what the algorithm was trained with causing it to slowly (or quickly) deviate from reality. An example would be training an autonomous vehicle in the Arizona summer, then having that vehicle drive in a Minnesota winter. The data could also be used in a feedback loop, where the outputs of the algorithm are used as an input to another algorithm. This can cause a problem to grow worse.
So how do we then make Responsible AI? We first need to understand all these potential issues and design in responsibility into the architecture, into the model, into the data. This has to be a conscious effort rather than a matter of fact. We need to have models that produce explainable outputs, and we must maintain data quality, over just concepts of Big Data at all costs. We need to work together as these issues are larger than any one person or organization. As Alphabet’s CEO Sundar Pichai stated, “There is amazing opportunities to be unlocked and so we want to take a bold approach to drive innovation, but we want to make sure we get it right. And so we want to be responsible in our approach. And so we think about our approach as being bold and responsible and understanding that framework and approaching it that way.”
A note on the picture, which was generated using Adobe Generative AI. The author used “Linocut” Art with the prompt: “Create an image of Benjamín Franklin writing “Haste makes Waste” in Poor Richard’s Almanack, in the year 1753”.